Executive Summary
- Companies rely on automation to optimize productivity and improve efficiency, and there is increasing demand for more advanced automation solutions.
- Automation technology is advancing through six generations — from robotic process automation to integrated ecosystem automation — with each generation building on the previous generation and having its own strengths and weaknesses.
- The latest generation, cognitive automation, combines human-like observation and adaptability, maximizing the effectiveness and potential of automation technology.
- Understanding the evolution of process automation provides a useful basis for companies to develop process automation strategies.
Introduction
Companies are constantly seeking ways to optimize their operations, reduce inefficiencies, and drive innovation. A staggering $1.8 trillion is spent each year in the US on repetitive, mundane tasks such as data entry, invoice processing, and report generation. Process automation has been the solution to this problem for many companies. It has been a driving force behind business innovation and the effective use of finite human resources. The demand for more advanced process automation systems capable of addressing a greater variety of more complex processes is growing. This increasing focus on process automation technology is being driven by rapidly changing customer needs, a dynamic and increasingly competitive business environment, labor and capital constraints, and the rapid pace of technological innovation.
This paper is intended for business professionals who are struggling with how to improve process efficiency and reduce costs at their company. It explores how process automation software has traditionally been applied to the productivity problem and how artificial intelligence (AI), in its various forms, is changing the way we should be thinking about what processes can be automated and how these processes can be automated. The goal is to contextualize the state of the art in process automation technology by comparing previous, current, and future generations of the technology. Understanding this progression will help businesses select an approach that best aligns with their growth and innovation objectives.
The Evolution of Process Automation
The original promise of process automation solutions was that they would improve business productivity, reduce labor costs, and free up human capital to focus on more creative, strategic, and high-value activities. These solutions have largely delivered on that promise for the most basic and repeatable tasks, such as payroll processing, customer complaint handling, and data transfers. The success of automating basic tasks quickly led companies to find ways to automate more complex and business-critical processes, such as insurance claims adjudication, supply chain optimization, and regulatory compliance in financial services. However, the first generation of automation technology had difficulties handling the variability, interface changes, decision-making, risk-taking, and reasoning required for such tasks.
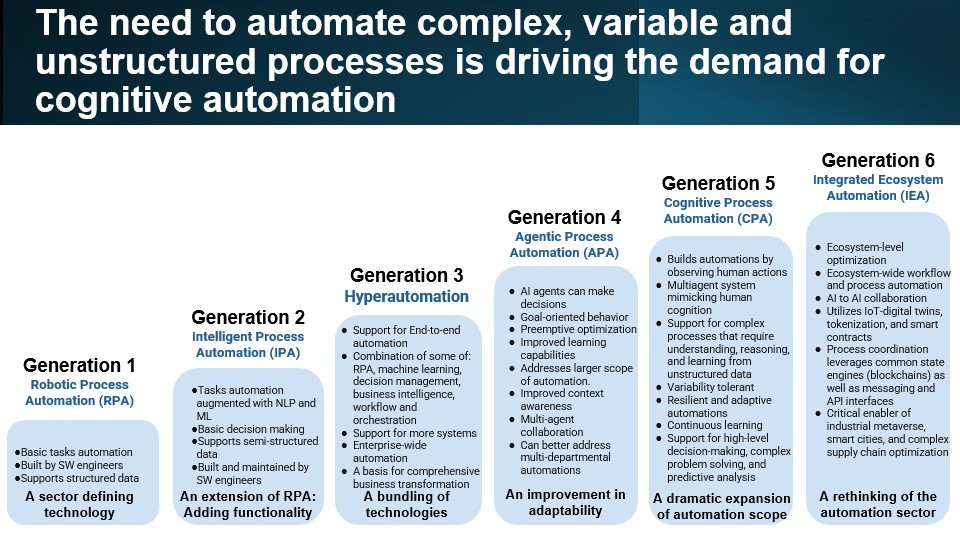
These challenges have been addressed to varying extents by successive generations of process automation technology, each of which builds upon the previous. With the integration of AI, process automation technology has become far more capable. Today, businesses rely on intelligent and cognitive systems that have transformed process automation platforms into essential business tools. The evolution of automation technologies (Figure 1) is a journey toward a more comprehensive reimagining of what businesses can achieve through automation. Let’s start our journey by looking at the first generation of process automation software: robotic process automation (RPA).
Generation 1: Robotic Process Automation
The first RPA systems emerged about 20 years ago. They operate primarily on structured data and have enabled the automation of the 10%-20% of basic and repetitive tasks. RPA uses digital workers (also known as robots or bots) to automate application execution and data movement at the user interface (UI) layer.
UI-level automation: Automating at the UI layer integration meant that automations were minimally invasive—they didn’t require reengineering or rewriting of underlying business code. RPA typically calls on external tools, such as optical character recognition, to facilitate document integration into processes.
Costly to build but high return: RPA automations were and still are typically designed and built by software engineers or trained business staff. As a result, these automations are expensive to build and maintain. Nonetheless, RPA has significantly reduced the need for manual work in processes like data entry and reconciliation in many industries, delivering significant savings.
Rules-based and variation-limited: RPA relies on a rules-based approach: automation developers, software engineers, or process experts must explicitly define what actions to take when changes occur that prevent a rule from executing correctly. This approach struggles when a process faces variations like changes in UI, screen resolution, or network latency—issues that are common when integrating external software-as-a-service (SaaS) solutions or deploying virtual desktop infrastructure. Such variability forces automation developers to frequently modify the rules to manage unexpected exceptions. As a result, these automations tend to be rigid and inherently fragile. Due to these limitations, RPA is poorly suited for processes that involve high variability, span multiple departments, or require judgment and decision-making.
Suited for simplicity: Some companies have moved past some of RPA’s limitations through significant customization and exception handling coding. However, these systems are usually brittle and rigid and require considerable maintenance. RPA is still fundamentally best suited to address relatively simple processes.
Key Characteristics of Robotic Process Automation:
- UI interaction automation
- Inflexible; requires strict rules-based processes
- Noninvasive integration
- Easy to scale
- Process-oriented
- Simple implementation
- Good return on investment for simple processes
- Cannot handle complex decision-making
- Needs constant updating as processes change
Example Use Cases for Robotic Process Automation:
INVOICE PROCESSING
A financial services company uses RPA to automate the extraction of data from invoices and input it into its financial systems for reconciliation, reducing manual errors and accelerating processing.
PATIENT DATA MANAGEMENT
A health care organization uses RPA to automate the transfer of patient data between systems, ensuring that health care providers have accurate information when they need it
Generation 2: Intelligent Process Automation
Intelligent process automation (IPA) emerged in 2017. It can automate more complex tasks and can be applied to approximately 30% of a typical organization’s processes. IPA extends RPA capabilities by incorporating tools such as natural language processing (NLP) and machine learning. This added intelligence supports basic decision-making, enabling the automation of processes that require some level of interpretation and decision-making, such as reviewing documents or emails, albeit within defined rules.
NLP capabilities: IPA can handle semi-structured data (such as emails and PDFs) and even some unstructured data (images, audio, and free-form text). IPA’s NLP capabilities are used in processes such as invoice scanning and contract analysis as well as customer support interactions through chatbots designed to provide more natural and human-like responses. NLP allows IPA systems to understand and process human language, which broadens the scope of processes that can be automated.
Improved adaptability: IPA goes beyond automating simple, static tasks; it introduces adaptability into automation. Machine learning models within IPA systems can learn from historical data, refining their performance over time and better managing variability. For example, machine learning helps NLP-enabled chatbots to interact with customers conversationally and provide personalized responses based on context and past interactions. This level of interaction would be impossible with traditional RPA. Beyond improving customer experiences, the use of machine learning allows IPA to reduce the risk of human errors and inconsistencies, improving the accuracy of compliance checking, data validation, and reporting.
Complex and costly: Implementing IPA is more complex and costly than RPA. It demands greater knowledge of machine learning models, substantial computational resources, and large volumes of accurate, representative data for training. Systems may still face difficulties with complex legal scenarios, human emotions, or ambiguous terminology, requiring ongoing monitoring and adjustment to ensure the models are working effectively. Ensuring that machine learning models do not reinforce biases or produce unreliable outcomes is also a significant challenge for IPA.
Key Characteristics of Intelligent Processing Automation:
- Machine learning and NLP integration
- Automation of complex, multistep processes
- Seamless integration with enterprise systems
- Enhanced decision-making capabilities
- Handles semistructured and unstructured data
- Adaptability through model refinement
- Dependence on high-quality data
- Limited understanding of nuanced contexts
Example Use Cases for Intelligent Processing Automation:
CLAIMS PROCESSING
An insurance company uses IPA to automate claim reviews by using NLP to analyze accident reports and related documentation. This speeds up claim resolutions while minimizing human involvement.
CUSTOMER SUPPORT AUTOMATION
A retail company uses IPA-powered chatbots to manage complex customer interactions, such as product recommendations or return processes, without the need for human agents. The chatbots enhance customer satisfaction by delivering timely and contextually relevant responses.
IPA has become an integral part of many business operations as advancements in NLP and machine learning continue. The ability to automate processes that involve human-like understanding and decision-making allows organizations to scale their automation efforts to previously unaddressed use cases and reduce reliance on manual oversight.
Generation 3: Hyperautomation
Hyperautomation involves combining multiple automation technologies to take a more holistic enterprise approach to automation. These include any combination of RPA, machine learning, workflow orchestration, business process management systems, and business intelligence. This approach enables organizations to automate complex workflows and integrate multiple systems, resulting in more cohesive, efficient operations across departments.
Broader and smarter automation: The bundling of automation and orchestration technologies allows organizations to automate a larger number of tasks and processes across departments and systems. It expands the scope and sophistication of automation and offers businesses a more comprehensive way to streamline operations and optimize decision-making.
Cross-department integration: Hyperautomation can support the integration of data and processes from different departments, such as human resources, finance, and the supply chain, resulting in more cohesive and efficient operations. This can improve collaboration across an organization and streamline tasks that require input or interaction from multiple business units.
Organization-wide optimization: Hyperautomation can dramatically reduce manual work in repetitive, high-volume tasks across departments such as IT operations. It can also optimize organization-wide resource allocation by analyzing and automating processes based on real-time data and historical patterns.
Complex and costly: Hyperautomation involves complex infrastructure setup; expensive, highly skilled technical personnel; and the coordination of multiple systems and departments, resulting in high upfront costs and maintenance efforts. Organizations may face increased risks associated with security and management challenges inherent in complex integrated systems, especially in cross-departmental automation initiatives.
Finally, there is a danger of “over-automation” when the degree of automation degrades customer experiences or process efficiency. In these cases, hyperautomation systems can become too rigid or might eliminate critical human oversight.
Key Characteristics of Hyperautomation
- Enterprise-wide automation
- Combination of RPA, AI, machine learning, and workflow orchestration
- Real-time data integration and analysis
- Cross-functional process automation
- High initial investment and maintenance costs
- Risk of over-automation and potential rigidity
Example Use Cases for Hyperautomation:
SUPPLY CHAIN AUTOMATION
A manufacturing company uses hyperautomation to optimize procurement, process orders, and manage inventory across a supply chain, which helps improve inventory visibility and reduce costs. Integrating various data sources and AI enables the company to make more informed decisions, reduce delays, and improve customer satisfaction.
END-TO-END LOAN PROCESSING
A bank uses hyperautomation to process loan applications—from document verification to approval—reducing the need for manual review and improving processing speed. This end-to-end approach helps ensure consistency, reduces bottlenecks, and provides a better customer experience.
Generation 4: Agentic Process Automation
Agentic process automation (APA) is an important augmentation of previous generations of process automation. It complements RPA and IPA rather than replacing them. Earlier systems were brittle and limited because they relied on predefined rules and machine learning models with supervised input. APA introduces AI agents: self-contained, autonomous software entities that, as the name suggests, have some degree of agency. These agents are designed to perform specific tasks or achieve defined objectives. This makes APA systems much more flexible and adaptable than systems built with previous generations of process automation technology. In theory, APA systems could automate as much as 60%-80% of an organization’s processes.
Goal-oriented actions: Like RPA and IPA, APA automations are usually coded by software engineers and trained software staff, albeit increasingly with the aid of generative AI. Unlike earlier automation systems, which were restricted to repetitive, rule-based actions, AI agents excel in managing tasks that require decision-making, context awareness, and dynamic interaction. These agents perceive their environment, process information, make decisions, and take actions to achieve their assigned goals.
Adaptable and proactive: Processes that are automated using APA are significantly more adaptable. They can adapt to changing contexts, collaborate with other agents or systems, and operate autonomously to drive outcomes. APA enables systems to act independently on behalf of an organization or individual, making decisions and executing actions based on predefined goals and evolving circumstances. AI agents are capable of collaborating with humans and other agents. By interpreting contextual information—such as physical sensor inputs, system data feeds, or digital data—AI agents respond proactively, not just reactively. They anticipate needs, identify opportunities, and autonomously initiate actions to achieve better outcomes.
Data-dependent and complex: Like many new technologies APA faces some challenges. The effectiveness of agents is highly bounded by the quality of the data they interact with and their ability to derive context from that data. Also, the autonomy of AI agents raises questions about accountability for decisions made by AI agents and addressing errors or unintended outcomes without immediate human oversight. Furthermore, integrating APA systems into legacy infrastructure can be complex, often requiring significant redesign and investment. Additionally, ensuring smooth collaboration between AI agents and human workers remains a challenge, particularly in environments where trust, transparency, and alignment of goals are critical for success.
Key Characteristics of Agentic Process Automation:
- Independent operations
- Goal-oriented behavior
- Proactivity
- Decision-making and reasoning
- Continuous learning
- Adaptability
- Development complexity
Example Use Cases for Agentic Process Automation:
DYNAMIC SUPPLY CHAIN ADJUSTMENTS
A retailer uses AI agents to autonomously monitor supply chain conditions, such as inventory levels, supplier performance, and external disruptions (e.g., weather or geopolitical events) as well as negotiate with suppliers, reroute shipments, and dynamically adjust production schedules to minimize delays.
PERSONALIZED CUSTOMER SUPPORT
A financial services company uses AI agents analyze customer data (e.g., spending patterns, past inquiries) to anticipate their needs and offer tailored financial advice or services. If a customer’s issue involves multiple departments (e.g., loans and investment accounts), agents collaborate to resolve the issue holistically.
APA is a major improvement over previous generations of process automation technology for some types of processes. By enabling systems to think, reason, and act at machine scale and speed, APA systems can handle complex, cross-functional, and evolving business environments. APA technology is a critical steppingstone toward true multiagent systems and cognitive process automation.
Generation 5: Cognitive Process Automation
Cognitive process automation (CPA) utilizes cognitive AI, a multiagent system that combines various AI capabilities to mimic human cognition. This enables CPA to perform traditional automation tasks faster and more robustly while also handling unstructured data, UI and runtime variability, and complex decision-making.
Observation-based intent recognition: CPA systems use advanced machine vision techniques to understand the layers and context of information on a screen or in a document. This enables CPA to observe human interactions with a UI, identify patterns, and go beyond recording the movement of screen artifacts to understanding the intent of user actions. By learning through observation, CPA eliminates the need for manually defining every step of a process, reducing the time and expense of extensive programming and maintenance. Automation systems built using this approach can quickly adapt to complex tasks that would otherwise require detailed coding and scripting. This is particularly useful in environments where tasks are difficult to formalize or describe through traditional programming methods. By capturing and analyzing human behavior, these systems can learn and replicate the subtle decision-making processes that often escape rule-based systems. For example, CPA can observe how an employee resolves an ambiguous customer inquiry by weighing context and past experiences and then replicate that judgment to manage similar cases in the future.
Context aware and self-correcting: Once process automations have been defined, CPA can derive context from data patterns, such as identifying common customer inquiries or frequently occurring exceptions. An understanding of context makes automations much more flexible and resilient. Automations can learn from experience and adjust themselves accordingly by modifying their decision-making logic or updating workflows to improve efficiency and accuracy. The adaptability of CPA results in flexible, self-correcting systems that reduce the need for human intervention.
Multiagent debate and best-result analysis: In CPA systems, multiple AI agents discuss possible solutions, evaluate options, and collaboratively select the most effective or accurate outcome. This leads to increasingly accurate and efficient process automation that can autonomously adjust workflows, refine decision-making rules, and modify approaches based on new data and experiences.
Highly adaptable: Because of its human-like adaptability and self-correction, CPA can address a much larger variety of complex processes than previous generations of process automation technology.
Potential risks: Depending on the level of reliance on machine learning and certain classes of agents, CPA systems can still suffer model biases, security vulnerabilities, and accountability challenges. These risks are minimized by CPA’s use of observational techniques, improved context sensing, advanced document processing methods, and multiagent debate. Risks that remain can be mitigated by implementing techniques such as bias detection audits and diverse training data sets to ensure fairness, accuracy, and security.
Weighing the benefits: The complexity of developing, deploying, and maintaining CPA systems—which often require specialized knowledge and sophisticated tools—must be carefully weighed against the benefits and efficiencies these systems deliver. While the upfront investment can be significant, the long-term cost savings and operational improvements often justify the effort.
Despite these challenges, CPA’s ability to continuously learn and adapt makes it an invaluable tool for organizations seeking to automate knowledge-intensive processes.
Key Characteristics of Cognitive Process Automation:
- Advanced NLP, machine vision, and semantic analysis
- Multiagent debate and best-answer mechanisms
- Self-learning and self-correcting
- Context-aware decision-making
- Handles unstructured data
- Human-like reasoning
- Potential machine learning model bias
Example Use Cases for Cognitive Process Automation:
CONTRACT REVIEW AND ANALYSIS
A law firm uses CPA to analyze legal contracts to identify risks and inconsistencies, while also continuously learning to improve its capabilities over time. This allows the legal professionals to focus on the more strategic aspects of contract negotiations.
PREDICTIVE NETWORK MAINTENANCE
A telecommunications company uses CPA to predict infrastructure failures, enabling proactive maintenance and reducing downtime. By analyzing data from various sources, CPA systems can predict potential issues before they escalate, helping to maintain service reliability.
CPA represents the convergence of advanced AI techniques and practical automation solutions, bridging the gap between structured task automation and human-like cognitive abilities. Its adaptability and ability to learn make CPA a powerful solution for addressing complex business challenges that require context-aware decision-making.
Generation 6: Integrated Ecosystem Automation
The future—and potentially most revolutionary—generation of process automation is integrated ecosystem automation (IEA). IEA takes automation beyond individual organizations by enabling ecosystem-wide automation, collaboration, and optimization. IEA is a combination of advanced cognitive process automation and next-generation digital infrastructure based on blockchain.
Trusted data fabric: Blockchain provides a common data fabric that all ecosystem participants can trust. With common trusted data, many other services become available as part of automations, including Internet of Things (IoT) sensors and actuators, which enable “digital twins” that link the physical world to the digital world. The new blockchain-based infrastructure will also support value transfer, tokenized reputation, decentralized governance, AI-to-AI collaboration, and “smart contracts”—self-executing agreements between parties without intermediaries.
Dynamic ecosystem optimization: IEA will transform industries by optimizing entire ecosystems, such as supply chains and digital industrial operations. It will also allow for real-time monitoring, analytic insights, and decision-making across an ecosystem. Organizations will be able to dynamically adjust operations based on current and anticipated conditions. For example, in a smart city, IEA could adjust traffic lights based on real-time data to optimize traffic flow, and a fashion house could adjust its spring line based on shifting patterns in consumer preferences and material availability.
Challenging and expensive: IEA will be difficult to implement both technologically and due to interorganizational governance, decision-making, and cost-sharing challenges. Potential approaches to overcome these challenges include fostering industry-wide collaboration, establishing clear governance frameworks, leveraging consortium-based models for shared infrastructure costs, and adopting advanced security solutions to ensure data integrity and compliance. Security risks and privacy regulation compliance will also be more challenging in multiparty environments, although solutions are being developed to address these problems.
Infrastructure needed for adoption: Implementation of IEA at scale will likely depend on the formalization of comprehensive digital infrastructure and standards at an industry or jurisdictional level. It will likely take a decade or more to fully realize the widespread potential of IEA, but efforts to implement the needed digital infrastructure are already underway in areas such as supply chain and smart cities.
The promise of IEA lies in its potential to create a seamlessly interconnected digital ecosystem, where information flows freely between organizations, and processes are coordinated across multiple stakeholders. Such an integrated approach can lead to unprecedented levels of efficiency, transparency, and responsiveness, allowing industries to operate as unified ecosystems rather than isolated entities.
Key Characteristics of Integrated Ecosystem Automation:
- Ecosystem-wide workflow and process automation
- AI-to-AI collaboration
- Use of IoT, digital twins, tokenization, and smart contracts
- Blockchain-based ecosystem-wide shared data
- Intercompany process coordination
- Critical enabler of complex industrial and supply chain optimization
Example Use Cases for Integrated Ecosystem Automation:
TRAFFIC AND TRANSPORTATION MANAGEMENT
Smart cities will likely use IEA with IoT, AI, and blockchain technologies to autonomously coordinate traffic signals, optimize public transportation, and manage emergency services.
AUTONOMOUS SUPPLY CHAIN ECOSYSTEMS
Manufacturing companies will use IEA with IoT digital twins, blockchain, and smart contracts to create a fully automated supply chain, coordinating everything from raw material sourcing to final product delivery across multiple companies.
IEA represents the culmination of automation evolution, bringing together the best of all previous generations and applying them at an ecosystem level. As the foundational technologies continue to mature, the potential for industries to achieve seamless, end-to-end automation across partners, suppliers, and customers becomes increasingly tangible.
Generations Summary

Conclusion
Process automation has become an indispensable tool for modern businesses. The technology has evolved considerably from early incarnations that required extensive and ongoing engineering effort and were limited to automating simple, repetitive tasks.
AI has radically altered the process automation landscape, allowing organizations to automate increasingly complex and variable processes. Agentic AI introduces goal-oriented decision-making, but it is the advanced techniques of cognitive automation that will be the most impactful. These systems are based on advanced cognitive AI that adapts to variability, learns continuously, and improves over time. These systems not only address the limitations of earlier systems, but they also make it possible to automate much more complex and variable processes.
As companies seek to future-proof their operations, they will look beyond automating simple processes inside departments to automating complex workflows that span multiple divisions and, eventually, even multiple companies. They will integrate, observational, cognitive, self-correcting systems with digital infrastructure that supports entire ecosystems. These systems will shape the next generation of industrial and digital innovation.
With each generation in the journey from RPA to IEA, automation has become more intelligent, adaptive, and capable of handling increasingly complex tasks. Businesses should prepare to embrace these advanced forms of automation to unlock new opportunities for efficiency, innovation, and growth. As digital ecosystems expand and collaborative technologies mature, the future of automation will be defined by interconnected systems that seamlessly manage both physical and digital worlds.
About the Authors
Douglas Heintzman is a cofounder and the CEO of Syncura
Hugh Frost is a cofounder and the Chief Product Officer at Syncura
Jet Theuerkauf is a cofounder and the Head of Customer & Partner Experience at Syncura
Daren Hanson is the Head of Sales & Marketing at Syncura
About Syncura:
Syncura is a leading provider of advanced cognitive document processing and cognitive process automation solutions. Its technology enables businesses to automate complex processes and handle dynamic document workflows with unmatched accuracy and efficiency. Its groundbreaking observational cognitive AI technology mimics human-like reasoning, allowing organizations to streamline operations, reduce costs, and improve productivity without extensive manual setup or retraining.
Syncura’s Cognitive Document Processor and Cognitive Automation Platform continuously adapt and learn, ensuring flexibility and scalability across industries such as finance, legal, healthcare, and others. With Syncura, businesses can confidently automate their most challenging processes and tackle complex document management tasks.
Syncura is committed to driving innovation in the automation space by combining cutting-edge AI technologies with deep industry expertise. By providing solutions that evolve with changing business needs, Syncura empowers organizations to stay ahead in an increasingly automated and interconnected world.